Demystifying the AI Black Box: Understanding the Inner Workings of Artificial Intelligence Systems
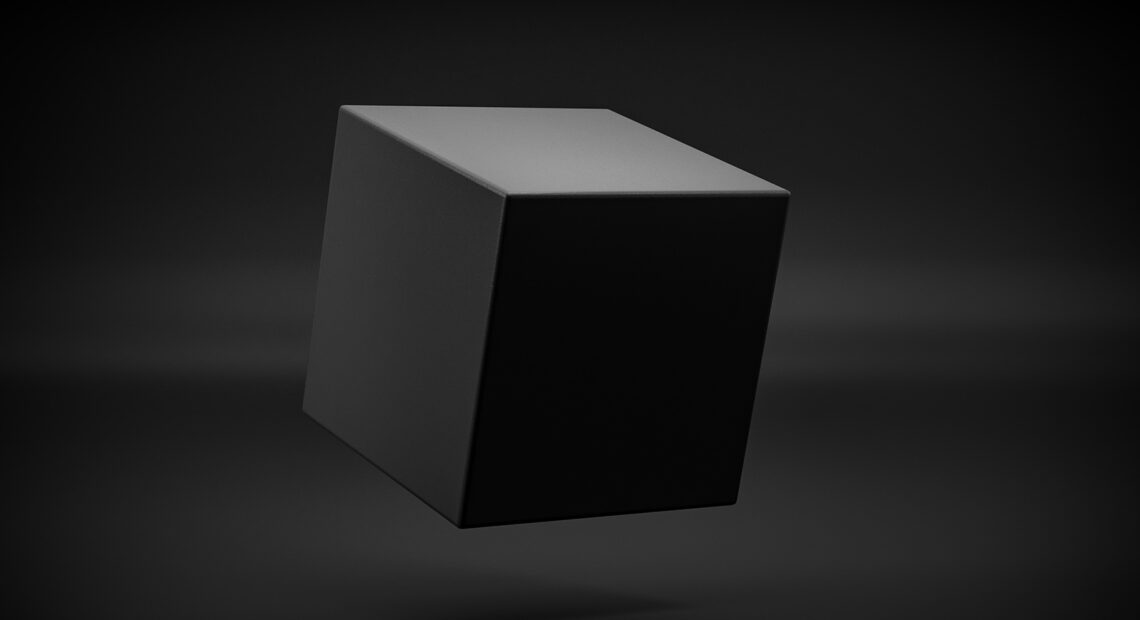
Artificial Intelligence (AI) has become an integral part of our lives, powering various applications and services. While AI algorithms can achieve remarkable feats, there’s often a lack of transparency regarding how they reach their decisions. This opacity has led to the emergence of the term “AI black box.” In this blog post, we will delve into the concept of an AI black box, exploring what it means, its implications, and potential approaches to address the issue.
Understanding the AI Black Box: An AI black box refers to an AI system or algorithm that provides output or decisions without offering clear insights into the underlying reasoning process. It essentially signifies a lack of transparency or interpretability in AI models, making it challenging for humans to comprehend the factors influencing the output. This lack of transparency is primarily prevalent in deep learning models, where complex neural networks make it difficult to trace the decision-making process.
Implications of the AI Black Box:
- Lack of Explainability: The foremost consequence of the AI black box is the diminished ability to explain how and why an AI system arrived at a specific decision. This poses challenges in critical domains like healthcare, finance, and law enforcement, where interpretability is vital.
- Trust and Accountability: Without transparency, it becomes challenging to trust AI systems. Users and stakeholders may question the reliability and fairness of the technology, impacting its acceptance and adoption. Additionally, in regulated domains, accountability becomes problematic as it’s difficult to ascertain whether the AI system adheres to ethical guidelines or biased decision-making.
- Bias and Discrimination: The opacity of AI black boxes can exacerbate issues related to bias and discrimination. If the decision-making process is not transparent, biases encoded in training data or unintended biases learned by the AI system can go unnoticed, leading to unfair outcomes or discrimination against certain groups.
Approaches to Address the AI Black Box:
- Model Transparency: Researchers are actively working on developing methods to enhance the interpretability of AI models. Techniques such as attention mechanisms, rule extraction, and model-agnostic interpretability methods aim to provide insights into the inner workings of black box models.
- Explainable AI (XAI): XAI is a field of study that focuses on developing AI models and algorithms that are inherently interpretable. By designing AI systems with built-in explainability, it becomes easier to understand the decision-making process and identify potential biases or errors.
- Regulatory Measures: Governments and regulatory bodies are recognizing the importance of AI transparency. Some jurisdictions are considering or implementing regulations that require AI systems to be explainable, auditable, and accountable. These measures aim to ensure ethical and responsible use of AI technology.
While the AI black box phenomenon presents challenges, efforts are underway to address this issue. The advancement of transparency techniques, the emergence of XAI, and the introduction of regulatory measures all contribute to making AI systems more understandable and trustworthy. By striving for increased transparency and explainability, we can harness the potential of AI while ensuring fairness, accountability, and the ethical use of this transformative technology.
Picture Courtesy: Google/images are subject to copyright